An organization owns a fleet of 20 aircrafts. The top 5 most problematic repairable LRUs in terms of failures are, U01, U02, U03, U04, U05. Any of the items fails on the aircraft, its mission is aborted.
Given that each aircraft is to operate for 100 hours for the next 1 year, the organization wants to anticipate the number of failures in order to facilitate their logistic support activities.
Total number of U01 is 25, 20 in the aircrafts, and 5 in the warehouse as standby spares.
Following is the snapshot of the failure data collected for those 9 units of U01 (serial number U01-01 to U01-09, while the remaining 16 units are not shown).
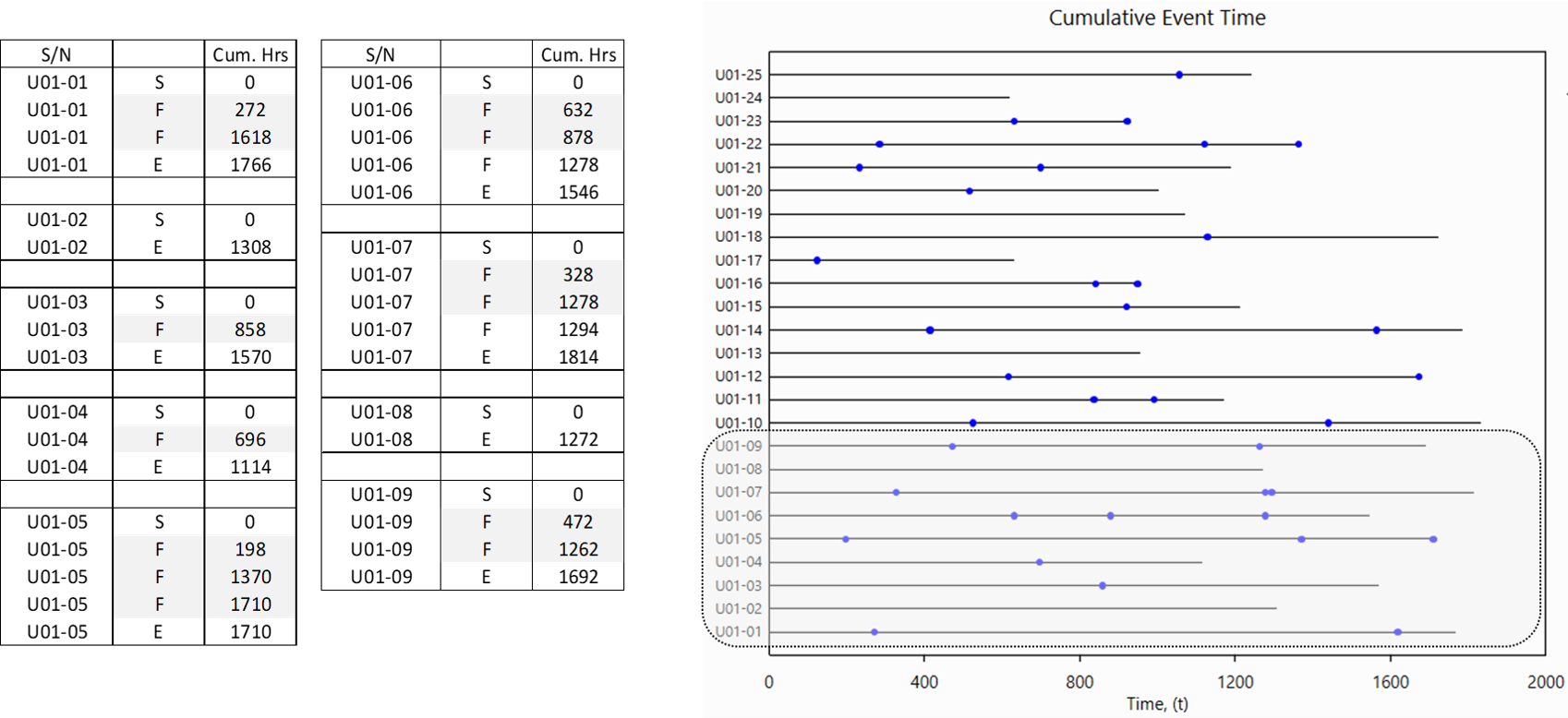
Take U01-01 for example, it has accumulated 1766 hours of operating time, and experienced 2 failures.
Failure data for U02, U03, U04, U05 also available in the same format (not shown here).
Given these historical data, we want to forecast the failures of these units for the next 100 hours of operations, so that the management can prepare the annual budget for these repairs.
Note that for repairable items, Life-Data Analysis is not applicable (unless the items can be considered as good as new after each repair).
Recurring Data Analysis (NHPP with Power Law model) is applied to each unit (U01 to U05). Following is a snapshot of the analysis for U01.
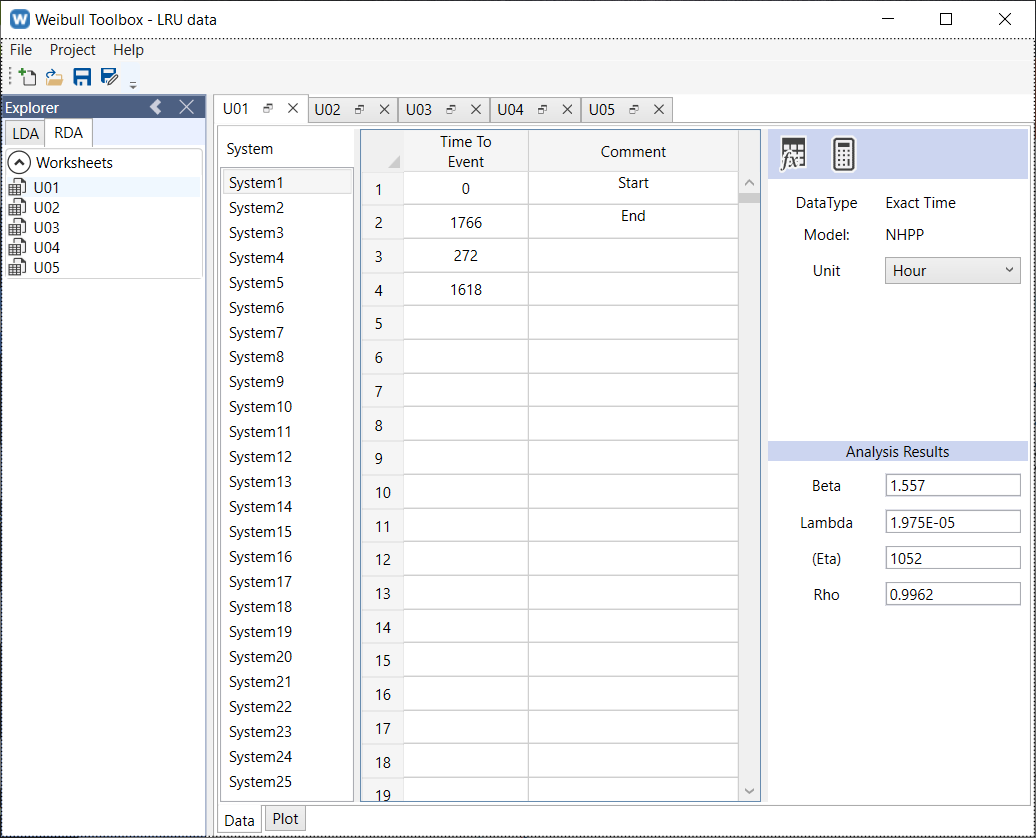
For repairable items (rotable spares), Life-Data Analysis is not applicable.
Beta and Lambda are the parameters of the mean cumulative function as shown.
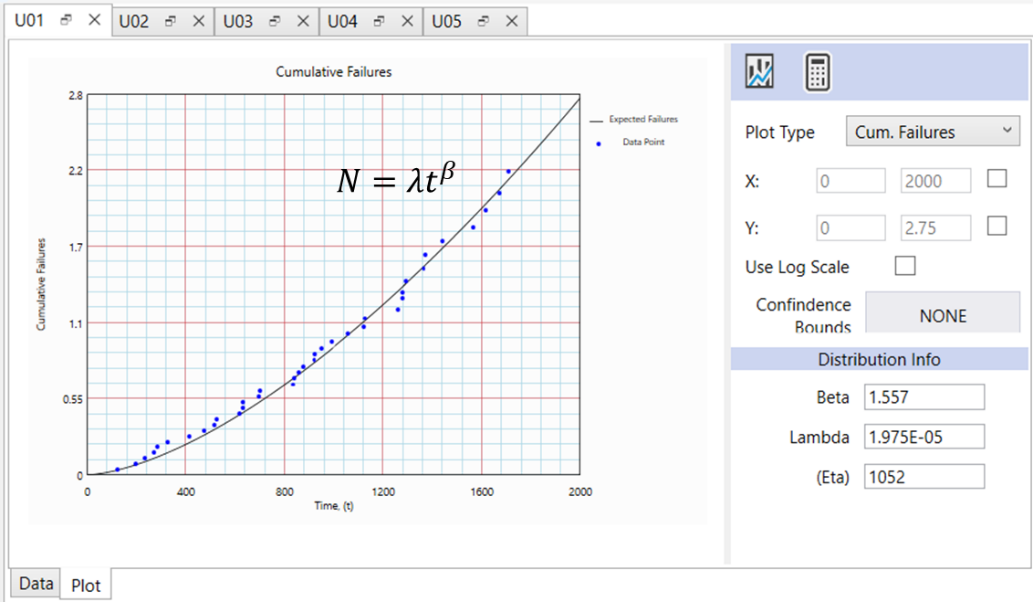
This function is used for projecting the number of failures in the future.
Query the model for Number of Failures at 1766 and 1866 hours respectively.
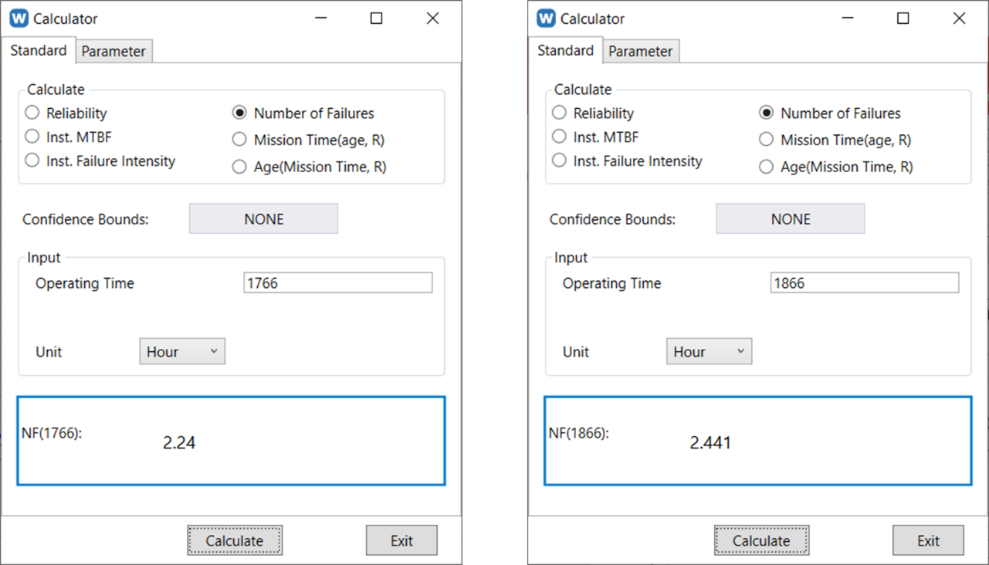
Expected number of failures for U01-0 for the next 1 year
(100 operating hours)
= 2.441 - 2.24 = 0.201
Similarly, the expected number of failures for the other U01 units are calculated.
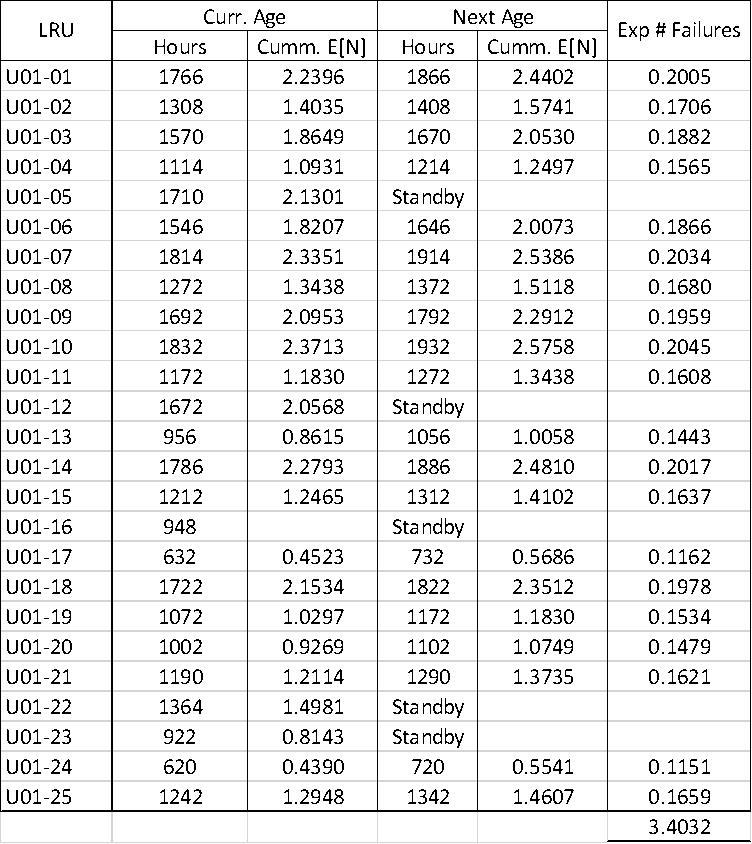
Repeat for the other LRUs (raw data are not shown here).
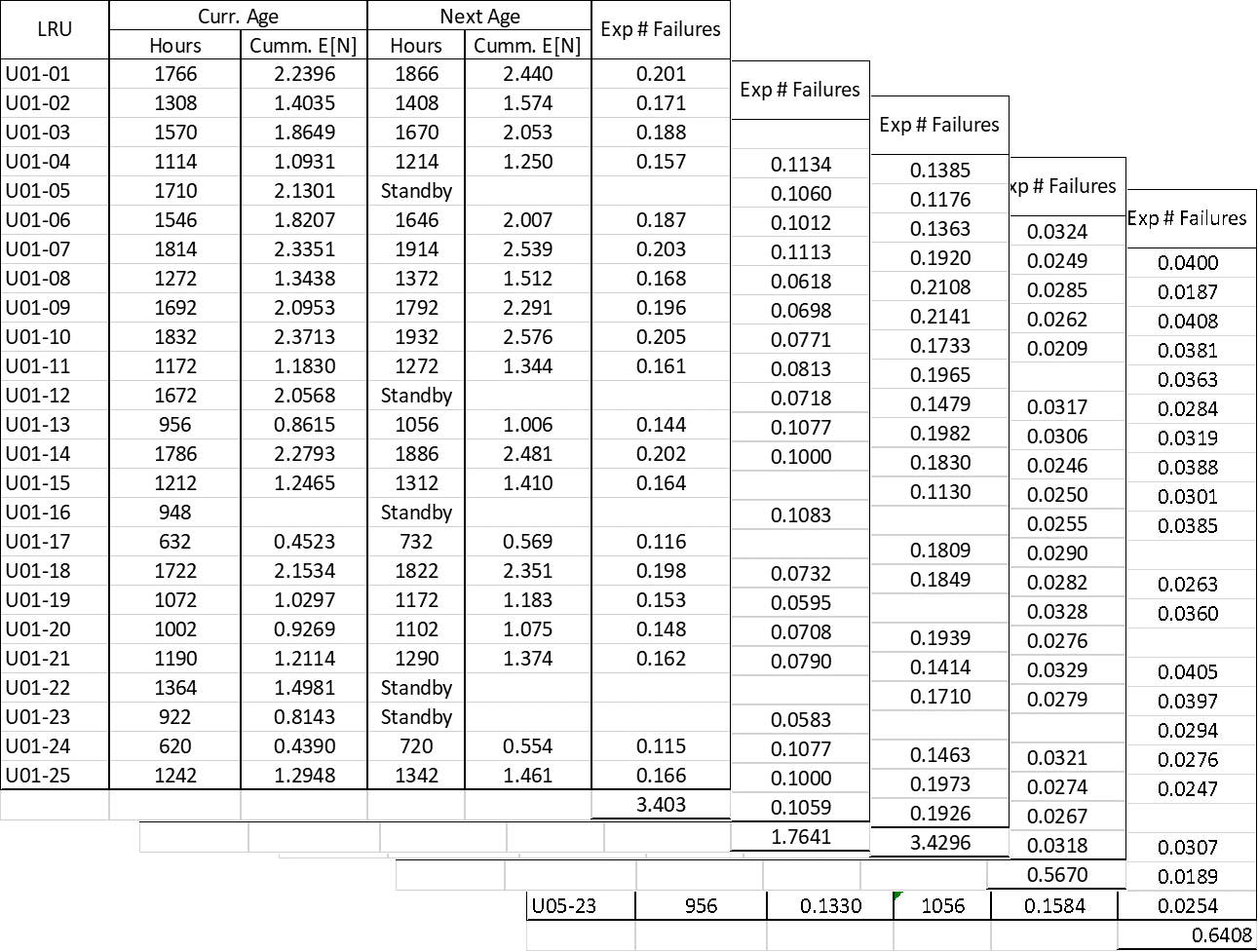
The expected numbers of failures for the following year for this fleet are as shown.
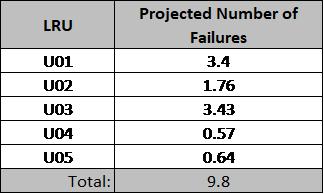
Conclusion
- Analyst should distinguish between repairable and non-repairable items. For repairable items, recurring data analysis applicable.
- Recurring data analysis can be used to project future failures for repairable items (rotable spare parts).
Comments
- Recurring Data Analysis can also be used to evaluate the failure intensity trends, and hence deduce the reliability of each units given its current age.
- Given that all of the items have increasing failure intensity trends, we can also deduce the economic life of each unit.
-End-