Author: Srikanth V Sura, CMRP, Principal Engineer, PETRONAS Carigali Sdn Bhd
Coauthor: Hongan Lin, Director, AssetStudio
Introduction
We consider an unmanned oil production setup with two pumps arranged in a standby configuration--one pump operates continuously while the other remains on standby. A switching controller is in place to automatically activate the standby unit if the operating unit fails. Figure 1 presents the reliability digital twin model for this system.
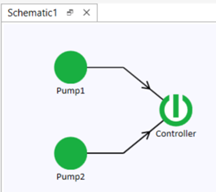
The failure of the switching controller itself does not directly impact system operation. However, it prevents the activation of the standby pump when required, potentially causing production loss. Since this type of failure is not immediately apparent, it is classified as a "Hidden Failure." For items with hidden failure modes, corrective maintenance (CM) for the controller is typically carried out during scheduled inspections or when the controller fails to perform its intended function—in this case, activating the standby pump when needed.
The following operating profile shows a scenario where the controller failure causes production loss.
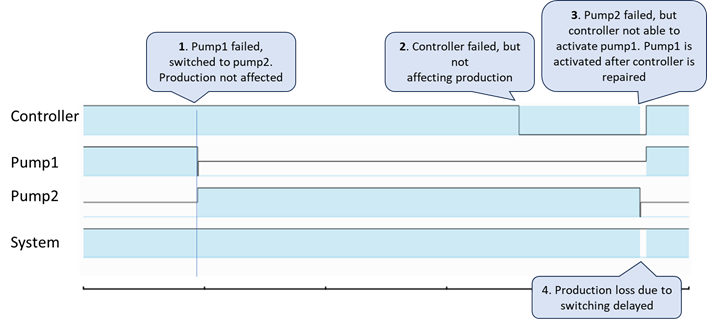
Note that upon failure of the controller, the system production is not immediately disrupted.
In our previous article, Distribution Analysis for Components with Hidden Failure Mode, we discussed methods to estimate the failure distribution of the controller and pumps using historical maintenance data. In this article, we focus on setting up a digital twin, as shown in Figure 1, to evaluate the production impact due to the controller unit's hidden failure mode.
Assumptions
- The controller unit is assumed to have a hidden failure mode, is non-repairable, and its operating life follows a Weibull distribution with β = 3.04 and η = 744 days. The two pumps are identical and repairable, with a constant failure rate and an MTBF of 0.75 year.
- Downtimes for the controller unit and pumps are set at 36 hours and 10 hours, respectively, using fixed downtimes for this demonstration.
- Repair/replacement cost for the controller unit is $50,000.
- Production is continuous (24/7) at 3,000 barrels per day, with each barrel valued at $70.
Cost Assessment of Current Run-to-failure Maintenance Policy
To simplify, we skip the digital twin setup details, except to note the use of a specialized construct called a "Startup Node" to represent the controller unit. The failure of this node does not impact normal operation but must function to activate an associated node (Pump 1 or 2) when switching from standby or post-maintenance.
First, let’s estimate the production loss over a 10-year period under the current run-to-failure policy, where the controller unit is only repaired when found to have failed. The following simulation, based on the digital twin model with 1,000 executions, provides an estimation.
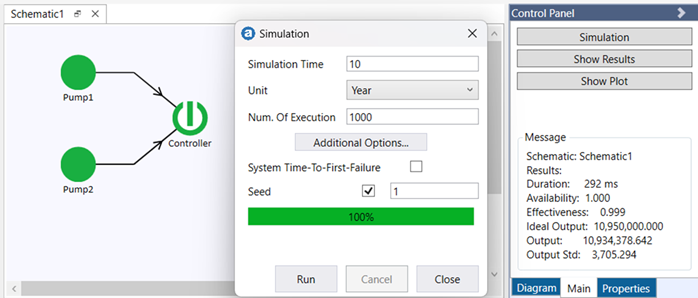
The simulated output is 10,934,378 barrels, compared to an ideal, failure-free scenario output of 10,950,000 barrels, resulting in a projected loss of 15,622 barrels. At $70 per barrel, this amounts to a total loss of $1,093,540 over the decade.
Below are the simulation results showing the various maintenance statistic of the controller unit.

The estimated number of controller replacements (CM tasks) over 10 years is 3.469 units, totalling $173,450 in replacement costs.
If we incorporate a preventive maintenance (PM) policy for the controller, can it help reduce production losses? If so, what is the optimal replacement time to minimize production losses?
Evaluating Cost-Benefit of Time-Based Preventive Maintenance
To evaluate potential cost savings from a time-based preventive maintenance (PM) approach, we consider a 2-year PM interval for the controller unit and rerun the simulation.
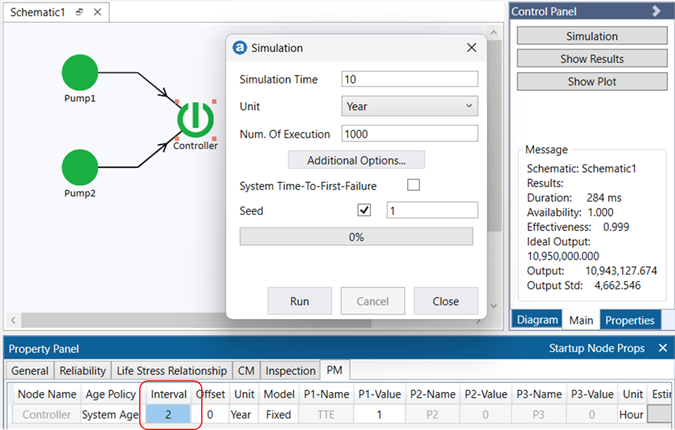
The output is 10,943,127 barrels, resulting in a loss of 6,873 barrels (valued at $481,110) over 10 years. This represents a nearly half-million-dollar improvement compared to the run-to-failure policy.

This time, we observe 1.525 corrective maintenance tasks and 4.103 preventive maintenance tasks over the 10-year span, with a total controller unit consumption of 5.628 units at a cost of $281,400.
We also conducted simulations using PM intervals of 1.5 years, 1 year, and 0.5 years, and summarized the results for all maintenance policies.
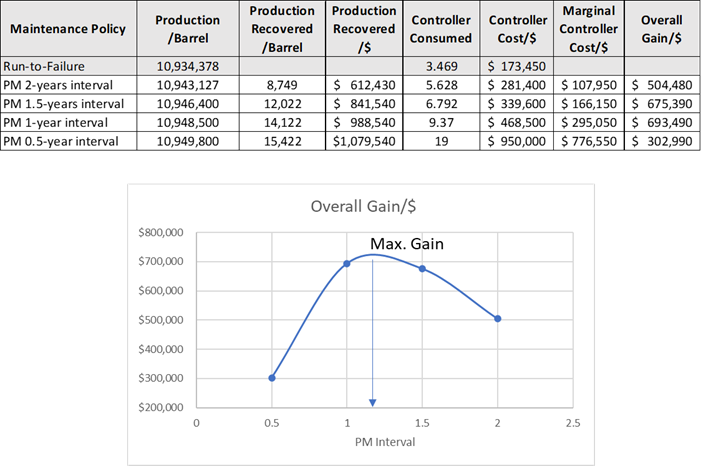
Note that the cost calculations in Figure 7 are based on the following assumptions:
- Ideal production is 10,950,000 barrels over 10 years.
- The value of each barrel is $70.
- Replacement cost for a controller unit is $50,000.
The Controller Cost column indicates the expenses incurred for controller unit replacements under each maintenance policy, while the Marginal Production Gain column represents the additional cost incurred with each maintenance policy.
Thus, for the 2-year PM policy, overall savings amount to $504,480, while the 1-year PM policy yields $ 693,490.
The plot in Figure 7 shows that the optimum PM interval is between 1 and 1.5 years.
Conclusion
This presentation illustrates how to create a reliability digital twin using the "Startup" construct to simulate hidden startup failures. With this construct, we can now quantify production impacts associated with the reliability and maintainability of components with hidden failure modes.
While this example primarily demonstrates the use and features of the "Startup" node construct, it also shows how to conduct a cost-benefit analysis by running simulations with different maintenance policies. This approach enables analysts to make data-driven decisions based on historical data, ultimately optimizing the organization’s bottom line.
-End-