Data Driven Decisions AeROS™
AeROS™Companies invest huge amounts in expensive data collection systems for their production facilities. Can we use these data to quantify the risks in the future? Can it be converted into information for improving production yields? AeROS™ provides a mathematical approach to convert historical data into useful information to achieve the desired production performance resulting in optimum financial health of your production assets.
Product Life Analysis Weibull Toolbox™
Weibull Toolbox™Weibull Toolbox™ is a statistical tool used to analyze and model the failure or survival characteristics of a product or system over time. It is often used in the field of reliability engineering, where the goal is to understand how and why products fail so that improvements can be made to increase their reliability and reduce the likelihood of failure.
Our Software
Using Historical data and Digital Twin to forecast production and financial impacts due to asset reliability performance
Our Consulting Services
Various reliability engineering consulting services for energy & manufacturing industry
Our Training
Our training courses focus on applying sound reliability engineering concepts to solve practical problems
About AssetStudio in 3 min...
Upcoming Training Courses:
Reliability Analysis for Repairable Systems (Course Outlines & Registrations)
- Location:Bali, Indonesia
Date:25 to 28 August 2025 (4 days)
Software Latest Updates:
Latest updates available for registered users:
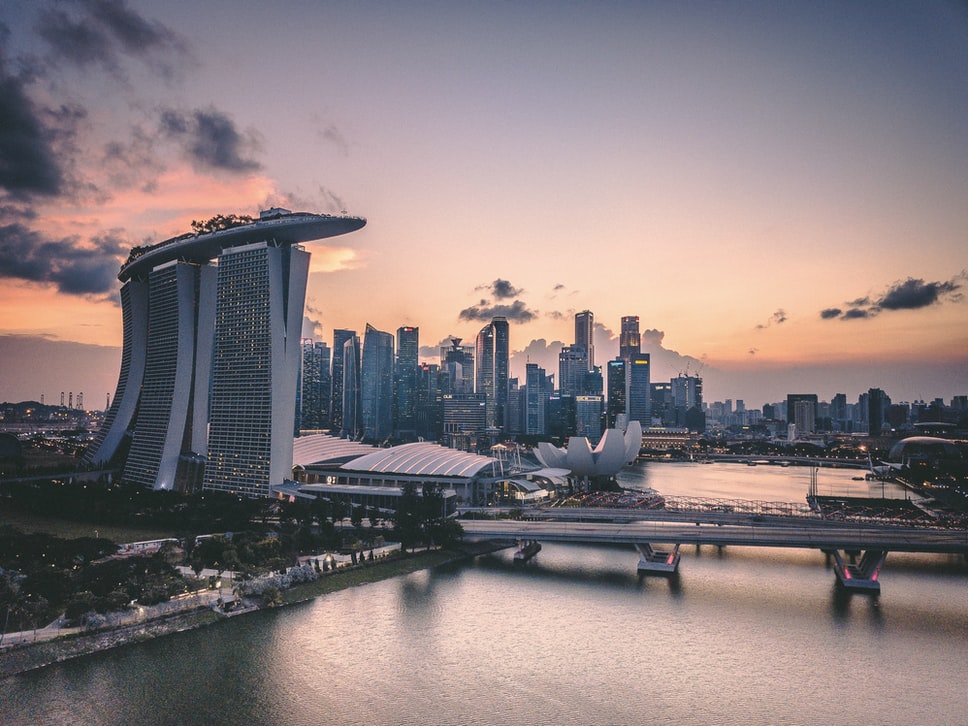
About Us - AssetStudio
Led by industry veterans with been there, done that experience, peace of mind is assured
- More than 50 years of combined consulting experiences
- Software tools developed by experienced reliability engineers
- Sound theoretical and practical competency in reliability engineering
- Highly competent in process and discrete manufacturing industries
We are here to empower the reliability community in their daily reliability analysis. To achieve that objective, we are dedicated to develop intuitive reliability software with impeccable customer service that is built on user-centric corporate philosophy.
Customer Base
Throughout the years, we have collaborated with numerous prominent companies, assisting them with their ongoing reliability analysis and CAPEX projects. Our enhanced comprehension of our customers has empowered us to swiftly transform industry challenges into improved software features. Furthermore, our extensive years of project experience have positioned us comfortably to tackle intricate technical issues with practical solutions.
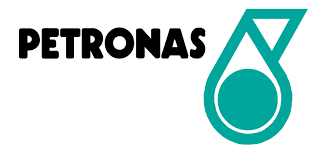
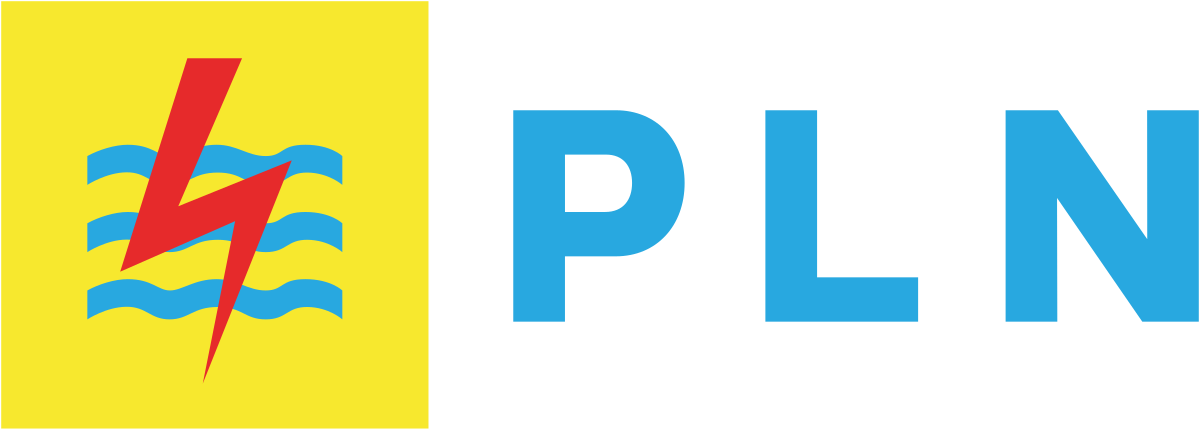
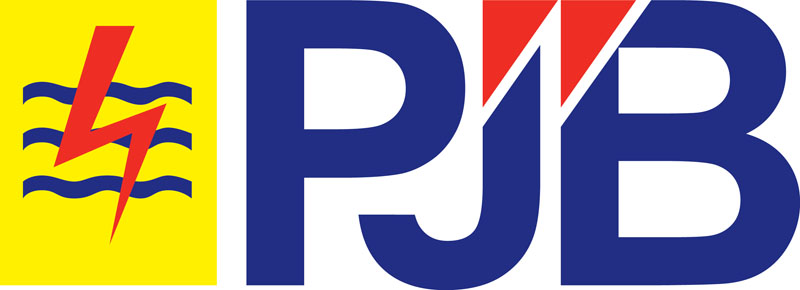
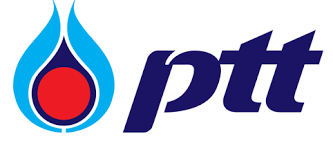
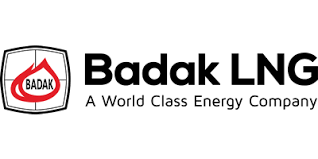
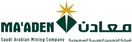

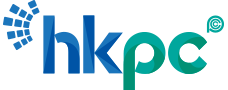
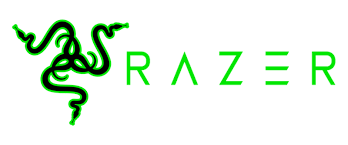
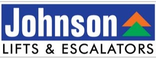
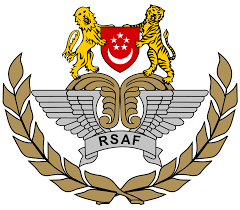
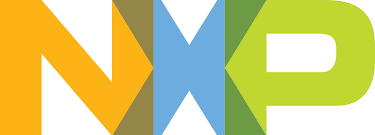
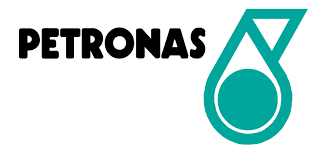
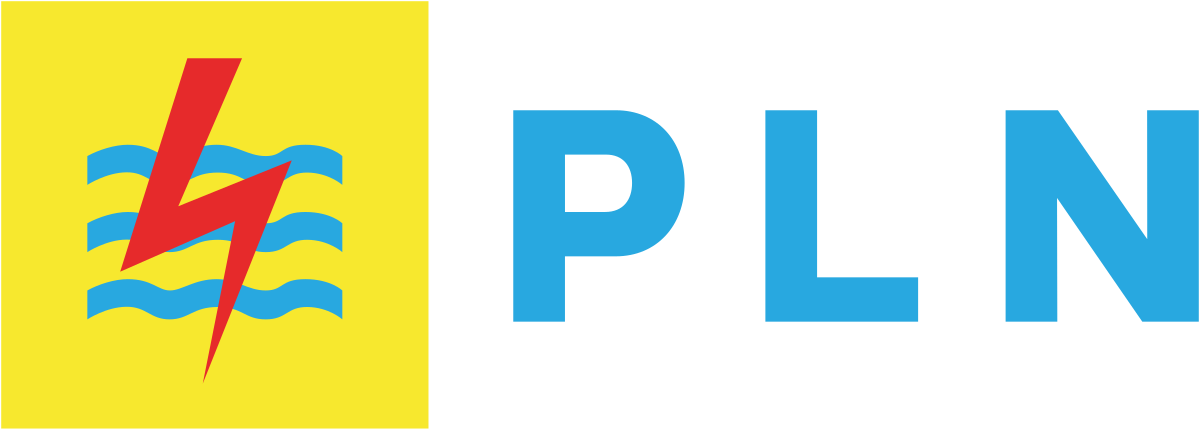
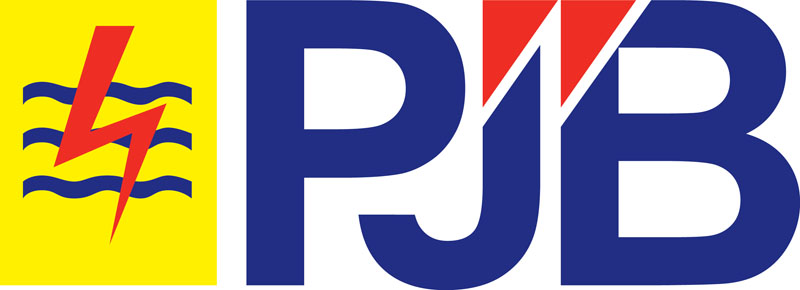
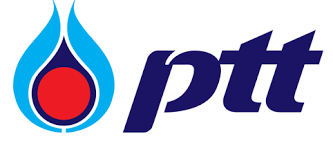
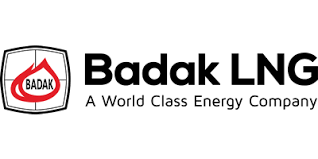
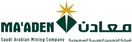

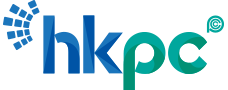
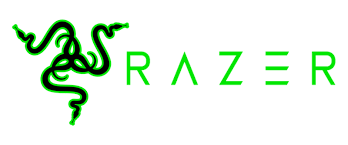
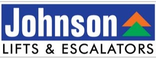
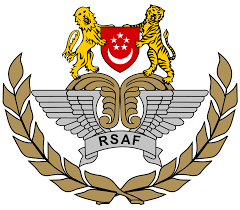
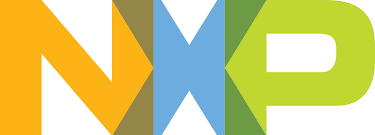
Testimonials
Customer centric engagement is the foundation and cornerstone of our success. We continue to strive for excellence and our satisfied customers have something to say about us below.
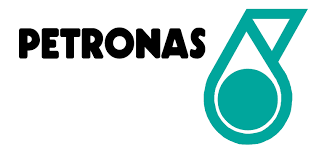
Principle Engineer
Petronas Carigali Sdn Bhd
" I am pleased to recommend the services of Asset Studio Pte Ltd (AssetStudio). Petronas Upstream has engaged AssetStudio to perform reliability analyses and conduct RAM (Reliability, Availability, and Maintainability) studies for our assets. "
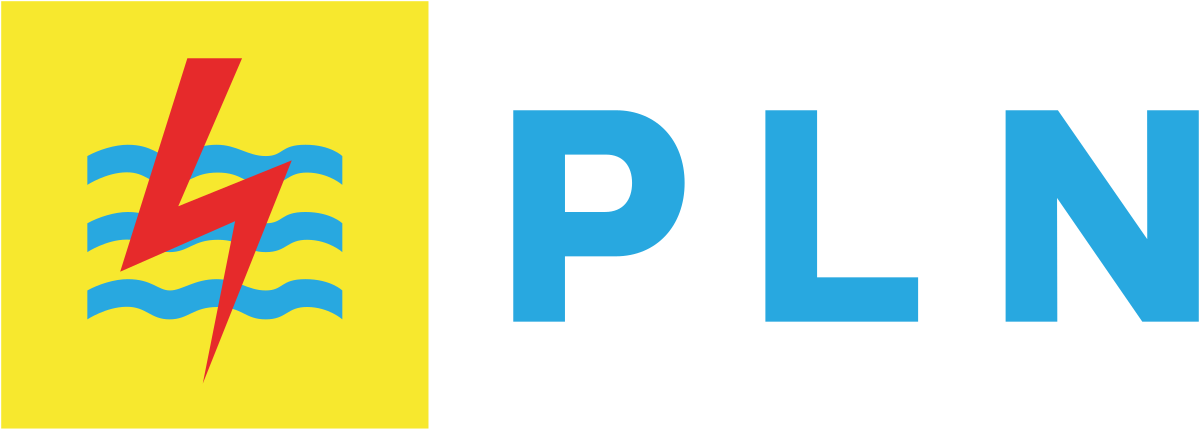
Project Manager
A Leading Power Authority in Indonesia
" We worked with AssetStudio on one of our CAPEX projects and were impressed by their flexibility and deep expertise in reliability engineering. I look forward to the opportunity to collaborate with them again in the future. "